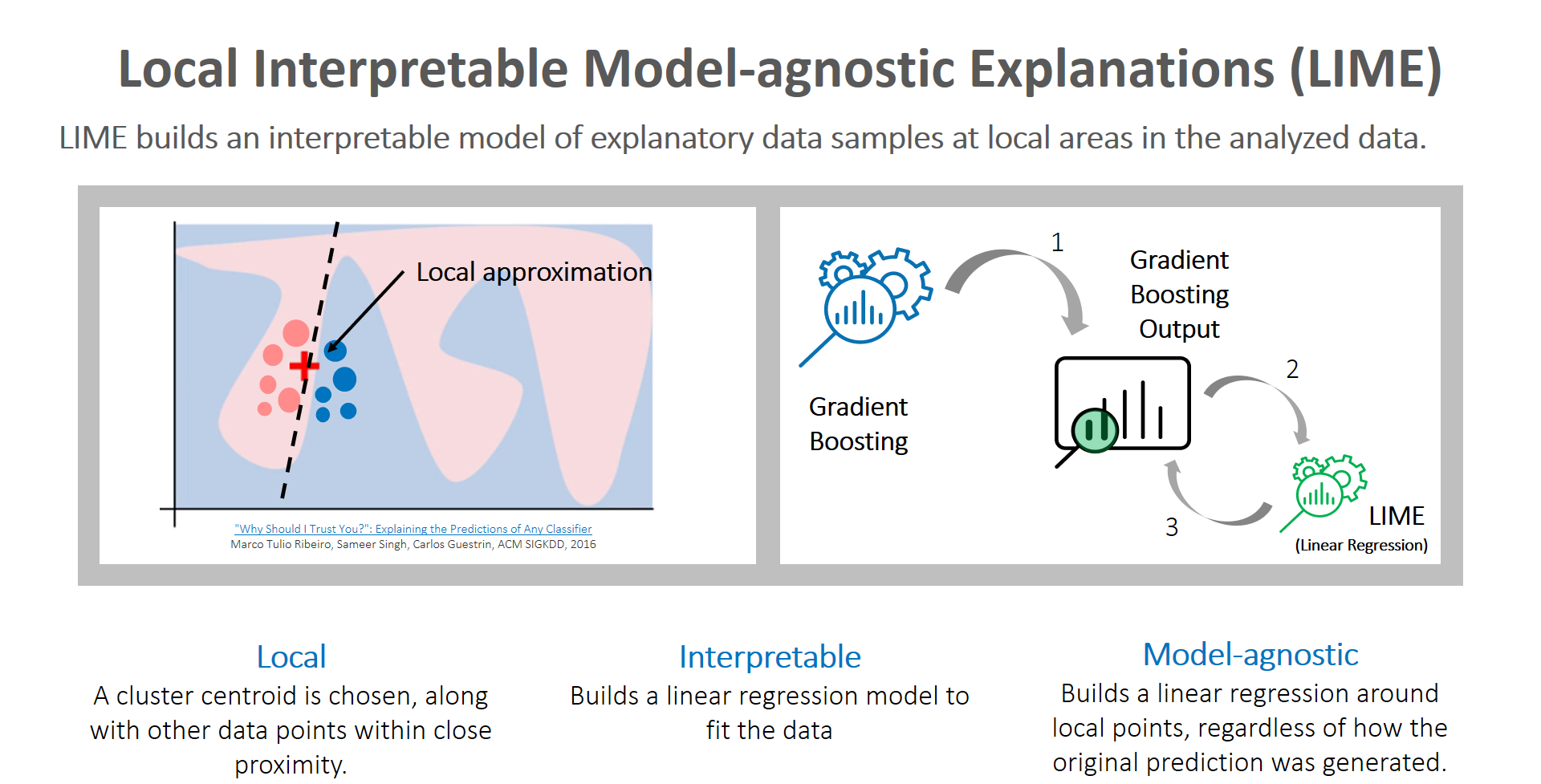
The acronym LIME, which stands for Local Interpretable Model-Agnostic Explanations, is a specific type of algorithm mode or technique that can help to address the black box program in machine learning.
At its most basic level, LIME will seek to interpret model results for human decision-makers.
One way to understand the use of LIME is to start with the black box problem, where in machine learning, a model makes predictions that are opaque to the humans using the machine learning technology. Visual models explaining the LIME technique show a "pick step" process in ML where specific parts of the data set are extracted to put under a technological microscope, to make them explainable by interpreting them for human audiences.
The "local" designation refers to a technique where the system chooses to interpret local results instead of global ones, because this is more efficient for the system.
The output of many LIME tools is composed of some chosen explanations of model activity in a given instance. This article uses the example of medical diagnosis to show how LIME can introduce specific components for a locally interpreted result, showing symptoms leading to a diagnosis.
In explainable machine learning and artificial intelligence, humans are better able to understand how the model is working, and ensure that it is working accurately. Without these types of tools, the black box model can lead to poor performance and outlier results that can be useless to practical utility.
0 Comments